Customer story
Verifiable Knowledge Base with Masdar
Verifiable Compute brings trust to another critical AI operation — Verifiable RAG.
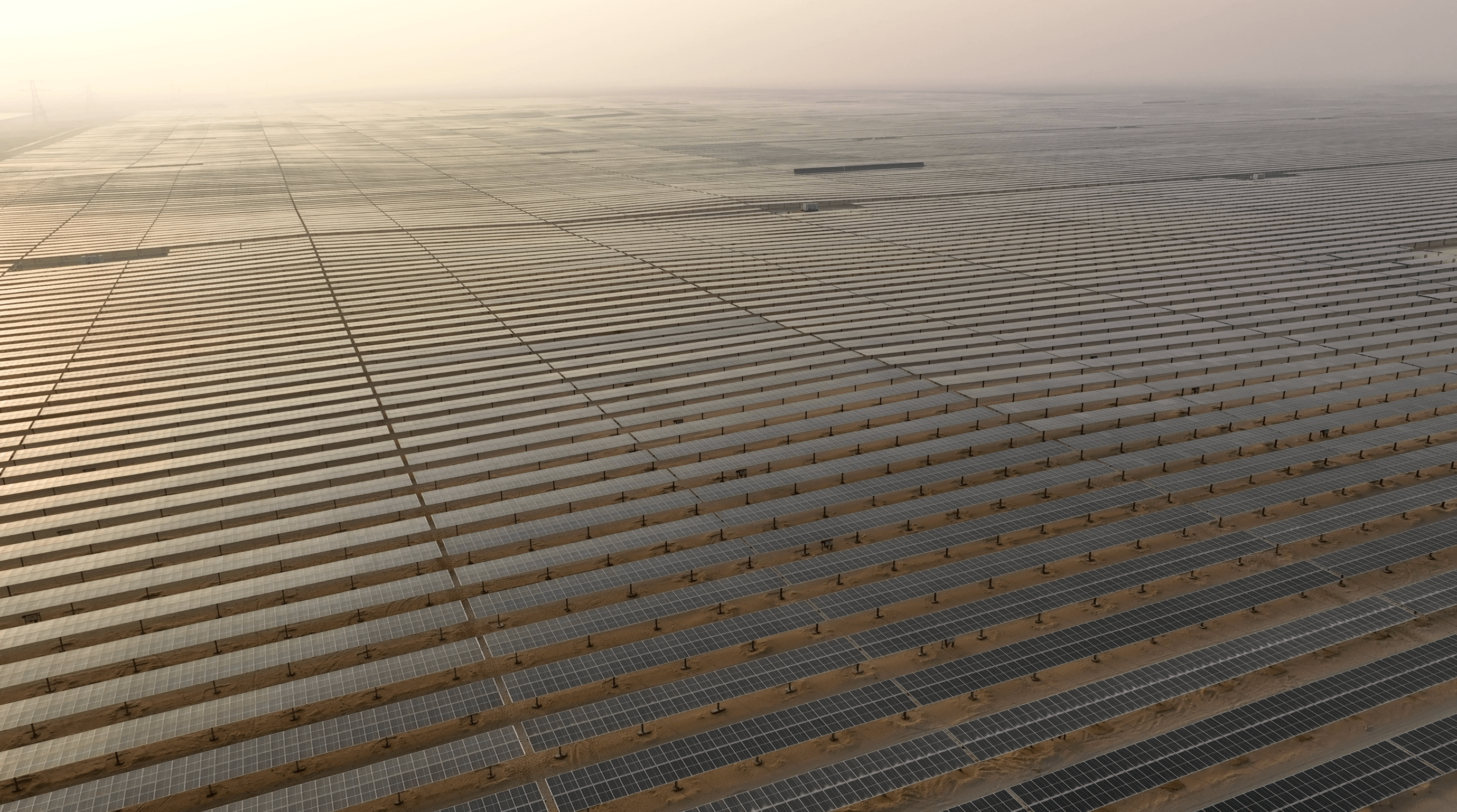
Key Takeaways:
Masdar implements RAG for pioneering energy research with accelerated insights.
EQTY introduces Verifiable RAG by creating tamper-evident records of RAG prompts.
The solution enables verifiable citations and compliance without exposing proprietary data or research.
A New Paradigm for Trust in RAG
Enterprises around the globe are integrating AI to drive more informed decision-making, and many are using retrieval-augmented generation (RAG) to ground specialized and curated information while deploying generalized LLM applications.
Masdar is one of the largest renewable energy investors in the world. It develops and operates clean energy projects across multiple continents, including solar, wind, and waste-to-energy facilities, while also investing in sustainable technologies and urban development projects.
As a pioneer in renewable energy technologies, Masdar sought to implement RAG to unlock new value from its quarterly technology journals that review the latest breakthroughs in green technology. While traditional RAG workflows could surface this type of institutional knowledge with ease, Masdar needed new ways to ensure any AI-driven insights were authentic, accurate, attributed, and aligned with critical policies.
To bring additional layers of trust to this workflow, EQTY Lab worked with Masdar to deploy its proprietary Verifiable Compute solution, a hardware-based cryptographic AI notary that isolates sensitive AI operations and creates a tamperproof record of the code computed in AI training and inference. With this framework in place, EQTY’s Verifiable RAG enhances traditional RAG queries by creating persistent, interoperable proofs of each prompt of the model.
For Masdar, these proofs of integrity could be used to confirm that:
The correct data was used to ground an inference.
RAG data could be verifiably cited without needing access to the proprietary dataset itself.
All RAG data remained in compliance with ESG policies and business objectives.
This capability is valuable in enterprise settings where decision-makers need to verify the accuracy and origins of AI-generated insights, ensure compliance with data governance requirements, and maintain accountability in automated analysis processes.
EQTY’s Verifiable RAG solution augmented a variety of important workflows for Masdar researchers, including accelerating impact analysis, assisting in purchasing decisions, and drawing from previous research while writing new journals.
As AI models and agents become increasingly multiparty, Masdar is positioning itself as a leader in establishing trust and confidence with policymakers, business partners, and consumers alike.
Masdar’s Priorities for AI-Driven Insights
Given the breadth of information Masdar researchers consider while evaluating clean energy solutions, RAG-enabled LLMs were an ideal technology to assist Masdar employees in traversing and summarizing their data-rich technical documents without compromising accuracy.
AI-driven citations can suffer from hallucinations, and models will invent references or misattribute information even when using real sources. This challenge is critical when dealing with technical documents and research papers, where precision and accuracy are essential for making informed business decisions. For organizations like Masdar operating in complex technical domains, ensuring the authenticity and accuracy of AI-generated citations is crucial for maintaining research integrity and trust in automated analysis.
With these challenges in mind, Masdar needed to transform how their employees access and trust insights derived from their technical journals. While AI could help synthesize this knowledge, Masdar mandated that every insight could be traced back to authentic sources.
To do so, Masdar and EQTY designed an AI system which:
Incorporated all of Masdar’s scientific journals into RAG.
Scanned for outdated data and refreshed it, when possible.
Contextualized alignment with international climate research, reports from other established agencies, and Abu Dhabi ESG policies.
Masdar and EQTY collaborated to develop a layered approach, one that took advantage of Masdar’s comprehensive research and data libraries to empower researchers to operate with new efficiency and confidence.
How Verifiable RAG Enhances Traditional RAG
Traditional RAG systems, while effective at retrieving relevant information, often operate as "black boxes" that make it difficult to verify how and why specific information was selected to inform an LLM's response. Verifiable RAG addresses this critical business challenge by providing cryptographically secured provenance chains — tracing and validating exactly which source materials and data points informed the LLM's output through tamper-evident linkages.
Unlike traditional RAG approaches that rely solely on similarity search without cryptographic verification of source linkages, verifiable RAG creates an immutable audit trail of precisely how information was retrieved and used, enabling organizations to cryptographically validate the entire inference chain from the retrieval of the source documents, the model that was deployed, to the final output.
This mathematically verifiable approach is essential for organizations making high-stakes decisions or operating in regulated industries where they must prove beyond doubt that AI-generated insights are firmly grounded in authorized source data, untampered with, and traceable through every step of the inference process — from initial retrieval through final generation.
The Verifiable RAG proof starts with verifiable creation of the embedding and metadata databases from data sources. The metadata database is indexed in a particular way that supports hash-addressing of specific data ranges, providing a Proof of Inclusion against a root hash, which can be used as a version identifier of the RAG corpus. Upon querying, prompt responses incorporate these verifiable referencing data, providing users with a highly granular certificate of authenticity for the response they receive.
In addition to acting as a digital notary, EQTY’s Verifiable Compute also provides real-time compliance checks and enforcement of AI business policies and new sovereign AI regulations such as the EU AI Act. Verifiable Compute’s new layer of trust is rooted right on the silicon of next-generation hardware from NVIDIA and Intel, setting the pace for a new standard for AI safety and innovation.
Looking Forward
In late 2024, RAG continues to be a cost-effective way for enterprises to keep their own LLMs current with new or proprietary information. Verifiable Compute adds a trust layer to this workflow by allowing anyone to verify the provenance of data considered in an inference, ensure compliance with regulations or policies, and maintain accountability in autonomous processes.
Presently, this benefit is crucial as enterprises integrate AI into their infrastructure. Verifiability will become mandatory as LLMs begin to interact with other LLMs and make decisions with limited human oversight.